Siddharth Swaroop
Machine Learning Postdoctoral Fellow at Harvard University.
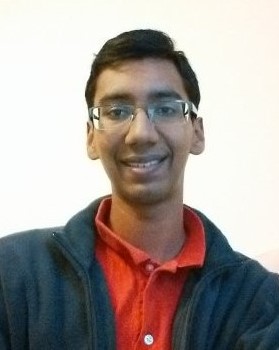
I am a postdoctoral fellow in the Data to Actionable Knowledge group, working with Prof Finale Doshi-Velez.
I obtained my PhD in the Computational and Biological Learning lab at the University of Cambridge, supervised by Prof Richard E Turner and advised by Prof Carl Rasmussen. During my PhD, I designed algorithms for large-scale machine learning systems that learn sequentially without revisiting past data, are private over user data, and are uncertainty-aware. My PhD was funded by an EPSRC DTP award and a Microsoft Research EMEA PhD Award. I also held an Honorary Vice-Chancellor’s Award from the Cambridge Trust.
Quick Links: Publications, Google Scholar, CV.
Research summary
My work focusses on understanding and adapting knowledge in machine learning models. This focus has two parts: designing new, more efficient, machine learning (ML) methods, and investigating the human and social impact of adapting ML models. More specifically, (1) I use a probabilistic framework to unify different knowledge-adaptation tasks, allowing me to design improved algorithms; and (2) I improve performance of human-AI teams by adapting ML systems to different humans in human-computer interaction (HCI), as well as translate AI policies into technical requirements for ML systems.
News
May 2025 | Paper published in ACM CHI Conference on Human Factors in Computing Systems (CHI 2025), Contrastive Explanations That Anticipate Human Misconceptions Can Improve Human Decision-Making Skills. |
Apr 2025 |
Paper at ICLR 2025, Connecting Federated ADMM to Bayes. Two papers at the Workshop on Building Trust in LLMs and LLM Applications at ICLR 2025, AI Companions Are Not the Solution to Loneliness: Design Choices and Their Drawbacks, and Rethinking LLM Bias Probing Using Lessons from the Social Sciences. |
Mar 2025 | Paper published in International Conference on Intelligent User Interfaces (IUI 2025), Personalising AI assistance based on overreliance rate in AI-assisted decision making. |
Dec 2024 | Three papers at the Workshop on Socially Responsible Language Modelling Research workshop at NeurIPS 2024, Understanding Model Bias Requires Systematic Probing Across Tasks, An Autonomy-Based Classification: AI Agents, Liability and learnings from the UK Automated Vehicles Act, and AI Agents & Liability – Mapping Insights from ML and HCI Research to Policy. |
Aug 2024 | Book chapter in “Towards Human Brain Inspired Lifelong Learning”, Chapter 4: Lifelong Learning for Deep Neural Networks with Bayesian Principles. |
Aug 2024 | Journal for Machine Learning Research (JMLR) paper published, Rethinking Discount Regularization: New Interpretations, Unintended Consequences, and Solutions for Regularization in Reinforcement Learning. |
Aug 2024 | Paper in American Medical Informatics Association (AMIA) Journal, Where do doctors disagree? Characterizing Decision Points for Safe Reinforcement Learning in Choosing Vasopressor Treatment. |
Jul 2024 | Paper at the Models of Human Feedback for AI Alignment Workshop at ICML 2024, AMBER: An Entropy Maximizing Environment Design Algorithm for Inverse Reinforcement Learning. |
Jun 2024 | Invited speaker at the 2nd Bayes-Duality Workshop in Tokyo, Japan. |
May 2024 | Paper published in International Conference on Intelligent User Interfaces (IUI 2024), Accuracy-Time Tradeoffs in AI-Assisted Decision Making under Time Pressure. |
May 2024 | Paper published in International Conference on Autonomous Agents and Multiagent Systems (AAMAS 2024), Reinforcement Learning Interventions on Boundedly Rational Human Agents in Frictionful Tasks. |
Apr 2024 | Gave talks at the SPIRAL Seminar Series (Northeastern University, USA) and TUFTS CS Colloquium (TUFTS, USA) on “Quick and accurate knowledge adaptation in machine learning”. |
Feb 2024 | Book chapter published in ‘Towards Human Brain Inspired Lifelong Learning’, Lifelong Learning for Deep Neural Networks with Bayesian Principles. |
Nov 2023 | Paper published in Transactions on Machine Learning Research, Improving Continual Learning by Accurate Gradient Reconstructions of the Past. |
Jul 2023 |
Paper at Advances in Approximate Bayesian Inference Symposium 2023, Improving Continual Learning by Accurate Gradient Reconstructions of the Past. Paper at Duality Principles for Modern ML Workshop at ICML 2023, Memory Maps to Understand Models. Two papers at the AI&HCI workshop at ICML 2023, Adaptive interventions for both accuracy and time in AI-assisted human decision making, and Discovering User Types: Mapping User Traits by Task-Specific Behaviors in Reinforcement Learning. Paper at Challenges of Deploying Generative AI Workshop at ICML 2023, Soft prompting might be a bug, not a feature. |
Jun 2023 | Invited speaker at the Bayes-Duality Workshop in Tokyo, Japan. |
Apr 2023 | Paper published in Transactions on Machine Learning Research, Differentially private partitioned variational inference. |
Dec 2022 | Organised the Continual Lifelong Learning Workshop at the Asian Conference on Machine Learning, 2022. As part of the conference, I also was a mentor in the Mentorship Program, chaired an invited talk session, and chaired a paper talks session. |
Jul 2022 | I have started as a postdoctoral fellow in Harvard University, with Prof Finale Doshi-Velez in the Data to Actionable Knowledge group. |
Jun 2022 | Invited talk at the Workshop on Continual Learning in Computer Vision at CVPR 2022 on “Knowledge-adaptation priors for continual learning”. |